When we think of agriculture, the image that often comes to mind is that of lush fields and busy workers. But there’s a silent revolution taking place in the background – a revolution that relies as much on pixels as it does on ploughs. Data labeling in agricultural technologies is not a peripheral process; it’s the very foundation upon which the future of farming is being built.
From precision agriculture to smart farming, the accuracy and granularity of data are directly linked to the efficacy of AI-driven applications in farming. For developers and AI engineers, this means the data labeling process needs to be meticulous and tuned to the unique needs of the agricultural sector. In this comprehensive exploration, we’ll dissect the various facets of data labeling as it pertains to agriculture, understanding its importance, the associated challenges, best practices, and real-world applications, as well as a vision of its future.
Introduction to Data Labeling and Its Role in Agricultural Technologies
The Backbone of AI in Agriculture
The agricultural sector has embraced AI with open arms. From drone-based surveillance to soil monitoring sensors, AI is transforming traditional agricultural practices into high-tech operations. But at the heart of these sophisticated systems lies a fundamental operation – data labeling. It’s the process of meticulously annotating raw data such as images, texts, and sounds, which is the starting point for machine learning algorithms.
Data labeling is particularly crucial in agriculture, where environmental conditions can be highly variable. Each crop, each field, and each region require tailored AI models, and this level of personalization is only possible with accurately labeled data. Whether it’s identifying crop diseases in early stages, predicting yields, or managing water usage effectively, AI applications heavily lean on data solidity to perform optimally.

The Importance of Accurate Data Labeling in Enhancing AI in Agriculture
Precision in Yield Prediction
Accurate data labeling is the linchpin in precision agriculture. In order to predict yields with high accuracy, AI models must be trained on data that is correctly annotated, representative, and free from bias. Quality data labeling can improve yield prediction models significantly, leading to efficient resource management and better decision-making for the farmer.
Disease Detection and Crop Monitoring
The ability to detect diseases early and monitor crop health via AI technologies is a game-changer for farming practices. However, for this to be effective, data labeling must be hyper-accurate. Infrared and multispectral imagery can read the invisible signals of a plant’s health, but this requires a high standard of labeling that captures nuances invisible to the human eye.
Water Management and Soil Health
In arid regions, optimizing water usage can mean the difference between harvest and famine. Similarly, maintaining soil health is a long-term investment in land fertility. AI must be fed with data that is clear and unambiguous in order to model complex processes effectively. This clear lineage of data quality starts at the labeling phase.
Challenges and Solutions in Data Labeling for Agricultural Use Cases
Diverse Data Types and Heterogeneity
Agricultural data comes in a myriad of forms – from satellite images of sprawling fields to the readings of a sensor buried in the earth. Labeling such diverse data is a complex task. Solutions such as multi-modal labeling tools and the use of domain-specific experts can help maintain data integrity across various types.
Labeling Bias and Its Remedies
Bias can creep into labeled data due to various reasons such as the preference of one crop over another or the selective labeling of defects. Leveraging inclusive and diverse labeling teams is a way to mitigate this. Tools that track and analyze labeling patterns can also help in ensuring that the data is not skewed in any particular direction.
Scalability and Consistency
With the increasing adoption of AI technologies, there’s a growing need for labeled data at scale. This immense volume must also be consistently labeled to avoid misinterpretations by the machine learning algorithms. Automated and semi-automated labeling tools can improve both scalability and consistency, making them critical weapons in a data labeler’s arsenal.
Best Practices in Data Labeling for Agricultural Technologies
Leveraging the Right Expertise
No one understands the intricacies of a field more than the field expert. In the context of agriculture, data labeling should involve experts who are well-versed in the domain. Pairing domain experts with machine learning experts can lead to a more informed and nuanced data labeling approach.
Continuous Quality Control
A standardized quality control process is pivotal in ensuring that the labeled data is of high quality. Implementing feedback loops, periodic reviews, and blind assessments can help maintain consistent quality, especially in high-stakes operations like agriculture.
Customizable and Agile Workflows
Ag in the 21st century is not just about using the latest tech, it’s also about being agile. Labeling workflows should be customizable to the specifics of the application and adaptable to changing needs. This can involve the rapid retraining of the labeling team, the use of flexible labeling tools, and almost instantaneous validation processes.
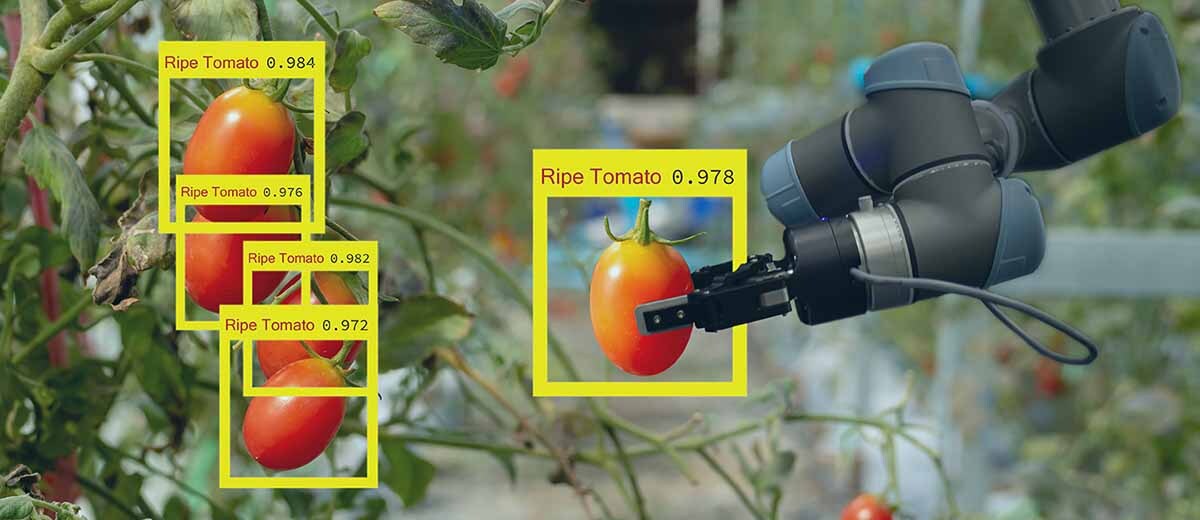
Case Studies: Real-World Examples of Data Labeling Improving Agricultural Processes
Drone-Based Monitoring and Accessibility
In the vineyards of California, drone-based monitoring systems have revolutionized data collection. These drones require accurate seasonal training data to pick up fluctuations that signify pest or disease outbreaks. Data labeling played a crucial role in the success of these systems.
The Green Revolution 2.0 in India
India’s green revolution 2.0, driven by data and technology, saw the use of AI in predicting crop health and advising on optimal harvest times. The government’s push for data collection and labeling as a public-private partnership was a significant factor in the success of these programs.
Future-Proofing Australian Farms
In Australia, the farming industry has been beset by the vagaries of climate change. By employing data labeling tools that account for these shifts, farmers have been able to stay ahead of the curve, making data labeling not just a process for today’s problems, but a solution for tomorrow’s.
Future Trends in Data Labeling for Agriculture and How Developers Can Prepare
The Rise of Machine Learning Operations (MLOps)
MLOps, like the better-known DevOps, focuses on efficiently deploying and managing machine learning models in production. In agriculture, MLOps can ensure that data labeling becomes a seamlessly integrated part of the workflow, reducing turnaround times, and speeding up model deployment.
The Integration of Blockchain for Transparency
Blockchain is being touted as the panacea for many data-related problems, and in agriculture, its application could lead to transparent and verifiable labeling. Imagine a world where the data used by an AI model for predicting yields can be traced back to the field it came from with irrefutable accuracy.
The Quest for Unsupervised Learning
The holy grail of AI is unsupervised learning, where an AI model can learn from data without the need for human intervention. Although this might be a few leaps away, the strides being made in collective intelligence and pattern recognition are certainly steering us in that direction.
A Call to Action for Agricultural Technologists and Developers
Data labeling is not just a technical process; it’s a foundational stone in the temple of agriculture’s technological future. It demands a conscious effort, a respect for detail, and a capacity for learning and adapting. For agricultural technologists and developers, the call to action is clear – invest in data labeling, not just as a precursor to your AI work, but as an integral and cherished part of it.
In conclusion, as we march towards a future where our fields are as smart as our devices, the role of data labeling in agriculture cannot be overstated. It’s the bridge between the real and the artificial, between the farms of today and the farms of the future. By understanding and prioritizing data labeling, we ensure that the bounty of tomorrow’s harvests is as fruitful and plentiful as the fields from which they spring.